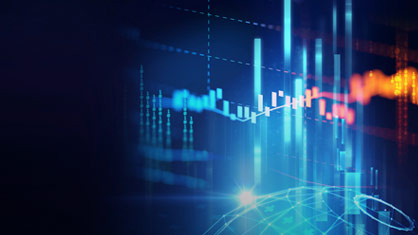
Analytics and Modeling
Learn more
Master's in Data Science
The integration of data science and business strategy has created a demand for professionals who can make data-driven decisions that move their organizations forward. Students can build the essential analysis and leadership skills needed for careers in today's data-driven world in Northwestern’s online Master of Science in Data Science program.
Northwestern Master of Science in Data Science (MSDS) students learn how to utilize relational, document, and graph database systems and analytics software built upon open-source systems such as R, Python, and Go, with TensorFlow and Keras for deep learning. They learn how to make trustworthy predictions using traditional statistics and machine learning methods.
START MY APPLICATIONRequest Information ATTEND AN INFORMATION SESSION
Focus your study in one of five specializations — Analytics and Modeling, Analytics Management, Artificial Intelligence, Data Engineering, Technology Entrepreneurship — or a track in general data science.
Students can further customize their studies with a wide range of elective courses, including:
The MSDS program features three languages: Python, R, and Go. Students in the data science master's program gain experience with these three languages and can tailor their studies to one language or another.
Learn why the master's in data science program has incorporated the Go Programming Language.
As a student in the master's of data science program, you'll learn how to:
The master's of data science program is offered in part-time and accelerated full-time formats.
Both master's of data science program formats offer a choice of specializations that enable you to create the course of study you need to meet your career goals. Whichever you choose, you will learn from experienced, engaged faculty who are thought leaders in their fields.
We welcome international students from around the world to apply for the accelerated MSDS option. If admitted to the accelerated option, international students are eligible to receive a student visa.
I learned the art of possibility, not just within the industry I worked in, but also about the power of understanding data science and using it to transform various industries in the world we live in.”
Sunny Yurasek, MS in Data Science ('20)
The Northwestern name and the predictive analytics aspect of the program have opened many doors for me.”
JR Runez, MS in Data Science ('16)
Courses range from statistical analysis to database systems to practical machine learning. Explore all the MS in Data Science online courses for full detail on the program's offerings.
To obtain the Master of Science in Data Science degree, students complete 12 courses that will enable them to build the high-level skills needed for careers in data science. Courses include core courses, specialization courses, a leadership or project management course, and a capstone project or thesis. Curriculum options vary slightly between the part-time and accelerated options.
REVIEW PART-TIME CURRICULUMREVIEW ACCELERATED CURRICULUM
A variety of factors are considered when your application is reviewed. Background and experience vary from student to student. For a complete list of requirements, see the admission page for SPS graduate programs.
Tuition for the Master's in Data Science program at Northwestern is comparable to other competitive online analytics programs across the nation. Financial aid opportunities exist for students at Northwestern. Complete details can be found on the tuition and financial aid for Data Science page.
Already accepted into the Master's in Data Science program? Get ahead and register for your classes as soon as possible to ensure maximum efficiency in your trajectory.
COURSE SCHEDULE & REGISTRATION REGISTRATION POLICIES & CONTACTS
As this interdisciplinary field continues to evolve, data scientists are defining new areas of focus to play key roles at various stages of the business cycle through modeling, engineering, and management. Consequently, professionals with expertise in data analysis, mathematics, machine learning, object-oriented programming, computer science, and business management are in demand across a wide range of industries.
MSDS faculty members include data scientists, professional researchers and consultants, social scientists, mathematicians, statisticians, and computer scientists who bring practical real-world experiences to the online classroom and engage with students on an interpersonal level. Most faculty members hold terminal doctoral degrees and have extensive teaching and business experience.
The MSDS Student Leadership Council strives to broaden awareness of data science in practice and build a sense of community in the program by fostering relationships among students, alumni, and leading industry professionals.
MS in Data Science faculty who teach courses in the Technology Entrepreneurship certificate and specialization discuss the many aspects of this field and share their own experiences and insights about startups.
Kimberly Chulis, PhD, has experience working and consulting for numerous companies and is the co-founder and CEO of Core Analytics® and the BrandMeter* Architect. Kim is an instructor of MSDS 470: Technology Entrepreneurship.
Bala Kamallakharan is the founder of Startup Iceland and also co-Founder of Dattaca Labs and Iceland Venture Studio. Bala teaches MSDS 472: Management Consulting and is developing an MSDS special topics course on product prototyping.
Dipyaman Sanyal has worked as a quantitative and financial analyst for numerous corporations and is the co-founder and CEO of dōnō consulting. Dipyaman teaches MSDS 474: Accounting and Finance for Technology Managers.
A master’s in data science from SPS can help you unlock a variety of in-demand career options across industries. Due to the ever-growing volume of data on everything from public health to consumer buying patterns, virtually every company and organization relies on experts in data science to make informed decisions and improve operations. In the online master’s in data science program, you’ll gain both theoretical and practical knowledge from industry experts in data science, mathematics, statistics, social sciences, and more, providing you with essential technical and analytical skills, and a competitive edge when applying to jobs. Whether you’re interested in finance, healthcare, e-commerce, or government, a data science master’s is the key to exploring the fields that interest you and leveraging that passion to create real-world change. For more information on the kinds of opportunities available to professionals with a master’s in data science, please visit our data science careers page.
Data science is a critical tool in making sense of the otherwise incomprehensible volumes of information all around us. By employing statistical analysis, machine learning, and data mining techniques, data scientists can identify trends, patterns, and correlations that might otherwise remain hidden. The pathways this creates helps businesses optimize their performance via consumer behavior and market research, manufacturers improve quality and efficiency, scientific organizations draw conclusions that enable innovation, and myriad other organizations optimize their performance. Data science is also an important tool for addressing social issues such as healthcare, education, and income inequality, as well as environmental change. Using insights from data scientists, policymakers and organizations can make informed decisions to benefit the greatest number of people. By earning your master’s in data science, you can become part of this force for progress and meet industry challenges head-on.
In order to be considered for the part-time online master’s in data science program, you must have a four-year U.S. bachelor's degree from a regionally accredited institution or foreign equivalent. A background that demonstrates strong quantitative reasoning skills is also preferred, but not required; our admissions committee considers all prospective master’s in data science students holistically based on the quality of application materials, academic records, and professional experience.
The online data science master’s program at SPS is designed to be completed within two to five years. Because most students balance their studies with full-time jobs, the average course load is one or two classes per quarter. This flexible part-time option allows you to apply at any time, begin during any quarter, and study at your own pace. If you are looking to fast-track your degree, consider applying to the in-person one-year data science master’s program.
If you’re feeling unsure about joining in the online data science master’s program, consider enrolling in a certificate program first. Northwestern’s School of Professional Studies offers certificates in Analytics and Modeling, Analytics Management, Data Engineering, Health Data Science, and Technology Entrepreneurship. With the stackable certificate option, you can complete a four-course program for graduate-level credit and later apply that credit toward your master’s in data science degree. The stackable certificate route to earning your MSDS is the best way to determine if the program is right for you while also building your resume with in-demand skills.